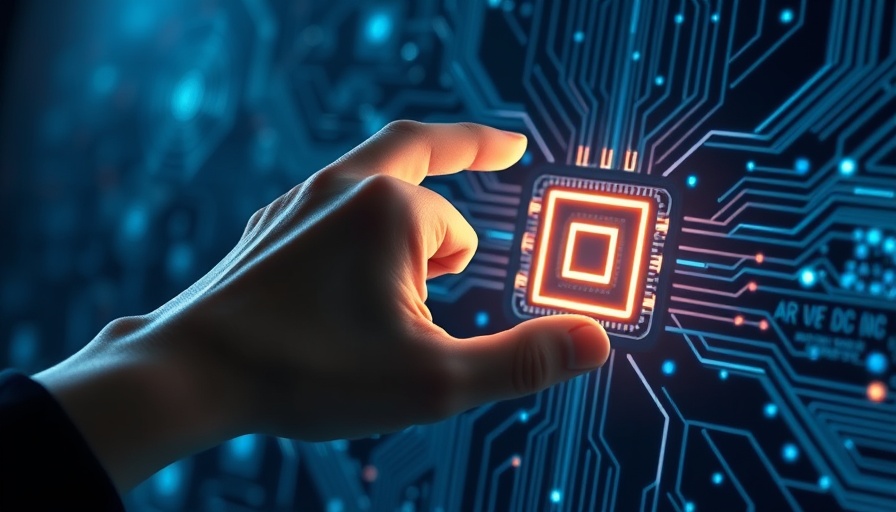
Understanding the Risks of Overtraining AI Models
In the rapidly evolving landscape of artificial intelligence, a new warning from researchers hailing from top US universities has made waves - extending the pre-training phase of AI models may not guarantee better performance. Instead, a phenomenon known as "catastrophic overtraining" may lead to diminished results, challenging the widely accepted belief that more data is inherently beneficial for training large language models (LLMs).
The Butterfly Effect in AI Training
The researchers, from institutions including Carnegie Mellon and Stanford, conducted a comparative study of the OLMo-1B model, scrutinizing two versions trained on 2.3 trillion and 3 trillion tokens, respectively. Surprisingly, the model trained with a larger dataset exhibited a decrease in performance by as much as 3% on significant benchmarks, such as AlpacaEval and ARC. This decline reveals a critical aspect of machine learning: too much training can lead to fragility.
What Is Catastrophic Overtraining?
According to the findings published in an academic paper available on arXiv, "catastrophic overtraining" occurs when models, after extended periods of pre-training, become increasingly sensitive to minor changes or noise. This heightened sensitivity can undermine previously acquired skills, potentially causing significant regression in model output. The authors introduce the term "progressive sensitivity" to describe this alarming trend, which inhibits models from adapting to new information during subsequent fine-tuning stages.
Identifying the Inflection Point
The analysis identified an essential concept known as the "inflection point," beyond which the risks of further training begin to outweigh the benefits. For many smaller models like OLMo-1B, this tipping point typically presents itself after training with more than 2.5 trillion tokens. The researchers caution that developers should not solely focus on increasing data volume but consider the potential internal instability that could emerge from excessive training.
The Future of AI Training
The implications of this research extend beyond mere computational techniques; they suggest a paradigm shift in how we approach AI model development. Rather than maximally increasing training data, a more balanced approach that considers optimal training durations and data quality is essential. The authors advocate for a renewed focus on model scaling that encompasses the entire training pipeline, emphasizing that in this case, sometimes, less truly is more.
A Cautionary Tale for Developers
For developers and AI practitioners, this serves as a poignant reminder: more data does not always equate to better performance. As the complexity of AI systems grows, understanding the inherent risks and limitations becomes crucial. This understanding can help bridge the gap between promising advancements in AI and the practical realities of model training. While pushing the boundaries of technology is essential, the findings highlight that caution and strategic consideration must be the guiding principles in AI model development.
As the landscape of AI continues to evolve, these insights could help shape new strategies that ensure models are robust, adaptable, and ultimately, effective in their responses and functions. Embracing this balance might unlock the next frontier in AI performance.
Write A Comment